Machine Learning: 2024's Breakthroughs and Discoveries
Machine Learning Dec 11, 2024 9:00:00 AM Ken Pomella 6 min read
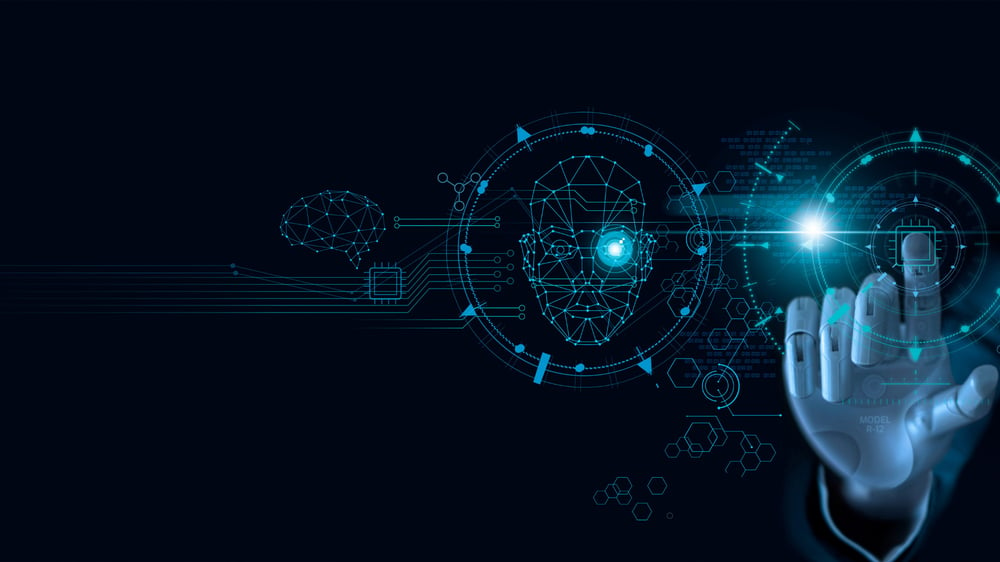
As we progress through 2024, the field of machine learning (ML) continues to make remarkable strides, impacting industries ranging from healthcare to finance, and setting the stage for new possibilities in artificial intelligence (AI). Fueled by advancements in computing power, data availability, and innovative algorithms, 2024 has already delivered breakthroughs that are transforming how we solve complex problems and interact with technology.
In this blog, we’ll dive into some of the most exciting breakthroughs and discoveries in machine learning this year, exploring their implications and potential for future innovation.
1. Generative AI Goes Beyond Text
Generative AI dominated the tech landscape in 2023, and 2024 is proving to be no different—except now, it's evolving beyond text-based applications. While large language models (LLMs) like OpenAI’s GPT and Google’s Bard remain central to the conversation, generative AI is making significant advancements in multi-modal applications, combining text, images, audio, and video.
Key Breakthroughs:
- Multi-Modal Models: New models like OpenAI’s GPT-V and DeepMind’s Gemini are seamlessly integrating capabilities for generating and understanding text, images, and video. These models can create realistic videos from textual descriptions or design interactive 3D environments.
- Generative AI for Music and Art: AI-powered music composition tools now allow users to create entire symphonies based on mood or theme. Similarly, tools like DALL-E 3 and RunwayML are empowering creators to design cinematic visuals and animations with minimal technical expertise.
Why It Matters: Generative AI is redefining creative industries, democratizing access to sophisticated tools, and enabling entirely new forms of storytelling. This shift is particularly significant for businesses leveraging content creation, advertising, and virtual reality.
2. Reinforcement Learning Takes on Real-World Problems
Reinforcement learning (RL), a subfield of ML focused on training agents to make decisions in dynamic environments, has reached new heights in 2024. No longer confined to gaming achievements like AlphaGo, RL is being applied to solve practical, real-world problems.
Key Breakthroughs:
- Robotics and Automation: RL is enabling robots to learn complex tasks like warehouse sorting, autonomous driving in unpredictable urban settings, and precision surgery with minimal human intervention.
- Energy Optimization: RL algorithms are being deployed to optimize power grids, reducing energy consumption and managing renewable energy sources more effectively.
- Finance and Trading: RL-powered algorithms are transforming quantitative trading by dynamically adapting to market conditions, outperforming traditional rule-based systems.
Why It Matters: Reinforcement learning’s ability to adapt and optimize in complex environments is unlocking efficiencies and innovations across industries, from logistics and manufacturing to renewable energy and finance.
3. AI Models That Explain Themselves
One of the biggest challenges in deploying machine learning at scale has been the “black-box” nature of many algorithms, where decision-making processes are opaque and difficult to interpret. In 2024, advancements in explainable AI (XAI) are addressing this challenge, making AI systems more transparent and accountable.
Key Breakthroughs:
- Interpretable Neural Networks: New architectures allow models to provide explanations for their predictions, offering insights into which features influenced the decision and how.
- Regulatory Compliance: Explainable AI is enabling businesses to meet stricter regulations, such as GDPR and AI-specific governance laws, which require transparency in automated decision-making.
- Healthcare Diagnostics: XAI models in healthcare are improving trust by showing clinicians why certain diagnoses or treatment plans are recommended, reducing errors and biases.
Why It Matters: Transparency in machine learning fosters trust, ensures fairness, and supports ethical AI development. As AI becomes embedded in critical domains like healthcare, finance, and law, explainability is essential for widespread adoption.
4. TinyML Expands AI’s Reach
While much of the focus in machine learning has been on larger, more complex models, 2024 has seen significant progress in TinyML—the application of ML on small, resource-constrained devices. From smart sensors to wearable devices, TinyML is driving a wave of innovation at the edge.
Key Breakthroughs:
- Ultra-Low Power Models: New algorithms optimize ML performance for microcontrollers, enabling real-time inference with minimal energy consumption.
- IoT Integration: TinyML is powering intelligent Internet of Things (IoT) devices for applications like predictive maintenance, environmental monitoring, and personalized health tracking.
- Edge AI Security: Advances in TinyML are enhancing security features in consumer electronics, such as on-device facial recognition and encrypted communication.
Why It Matters: TinyML is democratizing AI by making it accessible on affordable, widely available devices. Its low energy requirements and edge processing capabilities are expanding AI’s reach to remote areas, industrial settings, and everyday consumer applications.
5. Synthetic Data Revolutionizes Training
The demand for high-quality, labeled datasets remains a bottleneck in many machine learning projects. Enter synthetic data, a game-changing solution that’s taking center stage in 2024. By generating artificial datasets that mimic real-world data, synthetic data is solving data scarcity and privacy challenges.
Key Breakthroughs:
- Data Augmentation for Rare Events: Synthetic data is helping train models for scenarios where real-world data is sparse or difficult to collect, such as rare disease diagnosis or autonomous vehicle edge cases.
- Privacy-Preserving AI: By generating synthetic datasets that retain the statistical properties of sensitive data without exposing actual details, organizations can train models while complying with privacy regulations.
- Scaling AI for Emerging Markets: Synthetic data is bridging the gap in regions with limited access to high-quality datasets, enabling more equitable AI adoption globally.
Why It Matters: Synthetic data is transforming industries like healthcare, automotive, and finance, accelerating AI development while addressing critical privacy and accessibility issues.
6. Federated Learning Gains Momentum
As data privacy becomes a top priority, federated learning—a decentralized approach to training ML models—has gained traction in 2024. This method allows models to be trained across multiple devices or organizations without sharing raw data, ensuring privacy while enabling collaboration.
Key Breakthroughs:
- Healthcare Collaboration: Hospitals and research institutions are using federated learning to train models on patient data across organizations, unlocking insights without compromising privacy.
- Cross-Industry Adoption: Industries like finance and telecommunications are adopting federated learning to collaborate on shared AI models while keeping proprietary data secure.
- Standardization Efforts: Efforts to standardize federated learning protocols are making it easier for organizations to adopt this technology at scale.
Why It Matters: Federated learning is addressing critical concerns about data security and collaboration, enabling AI innovation in regulated industries and distributed environments.
7. Sustainability in Machine Learning
As the environmental impact of large-scale AI training comes under scrutiny, sustainability has become a key focus in 2024. The machine learning community is taking steps to reduce the carbon footprint of model development and deployment.
Key Breakthroughs:
- Energy-Efficient Models: Researchers are developing lightweight architectures and optimizing training processes to minimize energy consumption.
- Carbon-Neutral AI: Cloud providers and AI labs are committing to renewable energy sources and carbon offsets for large-scale training operations.
- Lifecycle Management: Best practices for model lifecycle management, including recycling and repurposing trained models, are gaining traction.
Why It Matters: Sustainable practices in ML are critical for balancing innovation with environmental responsibility, ensuring that the benefits of AI do not come at an unsustainable cost.
8. Cross-Disciplinary ML Research
2024 has seen a surge in cross-disciplinary research, where machine learning is combined with other scientific fields to drive innovation. These collaborations are breaking traditional silos and applying ML to solve complex problems.
Key Breakthroughs:
- Drug Discovery: AI-powered simulations are accelerating the discovery of new drugs by analyzing molecular interactions at unprecedented speeds.
- Climate Modeling: Machine learning is being integrated with climate science to improve predictions of extreme weather events and support sustainability efforts.
- Quantum Machine Learning: Advances in quantum computing are enabling new algorithms that outperform classical methods in specific applications like optimization and cryptography.
Why It Matters: Cross-disciplinary research is unlocking new applications for ML, pushing the boundaries of what AI can achieve in science, engineering, and beyond.
Conclusion: 2024 and Beyond
The breakthroughs and discoveries in machine learning this year are setting the stage for an exciting future. From the rise of multi-modal generative AI to advancements in federated learning and sustainable practices, 2024 is a year of innovation that emphasizes the versatility and transformative potential of machine learning.
As these trends continue to evolve, businesses, researchers, and developers have an unprecedented opportunity to harness the power of ML to solve real-world problems, create new technologies, and drive meaningful change across industries. Whether you’re an AI enthusiast, a data scientist, or a business leader, staying informed about these breakthroughs is essential for navigating the future of machine learning.
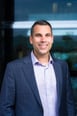
Ken Pomella
Ken Pomella is a seasoned software engineer and a distinguished thought leader in the realm of artificial intelligence (AI). With a rich background in software development, Ken has made significant contributions to various sectors by designing and implementing innovative solutions that address complex challenges. His journey from a hands-on developer to an AI enthusiast encapsulates a deep-seated passion for technology and its potential to drive change.
Ready to start your data and AI mastery journey?
Visit our Teachable micro-site to explore our courses and take the first step towards becoming a data expert.