Collaborative Machine Learning Projects: Success Stories
Machine Learning Nov 13, 2024 9:00:00 AM Ken Pomella 7 min read
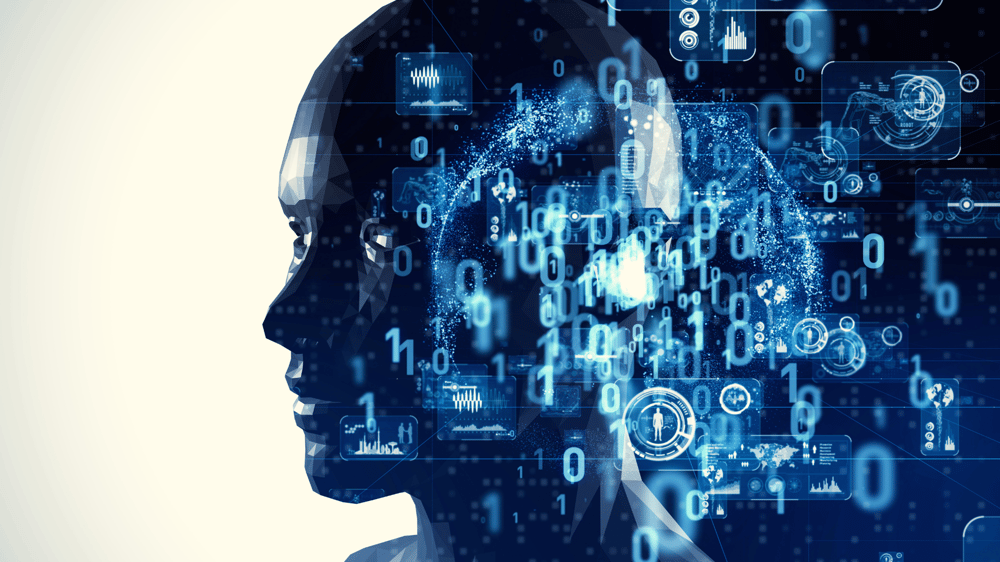
In the world of machine learning (ML), collaboration is often the key to success. Some of the most impactful ML projects have been the result of collaborative efforts, where teams of data scientists, developers, researchers, and organizations come together to solve complex problems and push the boundaries of what’s possible. Whether through open-source communities, industry partnerships, or cross-disciplinary teams, collaborative machine learning projects have led to breakthroughs in fields ranging from healthcare to environmental conservation.
In this blog, we’ll explore several success stories that demonstrate the power of collaborative machine learning. These projects showcase how diverse expertise, shared resources, and a unified vision can produce innovative solutions that have a meaningful impact on the world.
1. ImageNet: Revolutionizing Computer Vision
The ImageNet project is one of the most influential collaborative efforts in the field of computer vision and deep learning. Founded by Dr. Fei-Fei Li and her team at Stanford University, ImageNet is an open database containing millions of labeled images that have been used to train machine learning models. The collaborative nature of ImageNet, which involved contributions from researchers, universities, and volunteers worldwide, has led to groundbreaking advances in AI.
How Collaboration Fueled Success:
- Crowdsourcing Annotations: To label millions of images, the ImageNet team enlisted thousands of volunteers through Amazon Mechanical Turk. This crowdsourced approach allowed the team to create a massive dataset that would have been impossible to build through traditional means.
- Open Competitions: The annual ImageNet Large Scale Visual Recognition Challenge (ILSVRC) brought together researchers from around the world to develop and test their models using the ImageNet dataset. These competitions fostered collaboration and friendly competition, leading to significant advancements in computer vision algorithms, including the development of revolutionary architectures like AlexNet and ResNet.
- Open-Source Community: By making the ImageNet dataset publicly available, the project invited collaboration from researchers, companies, and institutions. This openness has helped accelerate innovation in fields beyond computer vision, as the techniques developed with ImageNet have influenced natural language processing (NLP), robotics, and more.
Impact: ImageNet’s collaborative approach has revolutionized computer vision, setting the standard for training image recognition models and driving the development of technologies like autonomous vehicles, facial recognition systems, and healthcare imaging tools. Its success shows how collaboration and open data sharing can lead to breakthroughs that reshape entire industries.
2. COVID-19 Open Research Dataset (CORD-19)
The CORD-19 project is a shining example of how collaboration in machine learning can address global crises. Developed in response to the COVID-19 pandemic, CORD-19 is an open dataset containing thousands of scientific articles related to the coronavirus. The dataset, created by a consortium that included the Allen Institute for AI (AI2), the White House Office of Science and Technology Policy, and other partners, aimed to empower researchers and ML practitioners to develop models that could extract insights from the vast body of COVID-19 literature.
How Collaboration Fueled Success:
- Interdisciplinary Partnerships: The project brought together government agencies, academic institutions, tech companies (like Microsoft and Kaggle), and researchers from diverse fields such as epidemiology, virology, and AI. This cross-disciplinary approach enabled rapid progress in understanding the virus, its spread, and potential treatments.
- Open-Source Platforms: By hosting the dataset on platforms like Kaggle, CORD-19 encouraged thousands of data scientists and machine learning engineers to participate in developing models that could analyze and synthesize information from scientific papers. Kaggle’s community of ML enthusiasts built various tools, such as NLP models to identify key research findings and track the evolution of the virus.
- Global Collaboration: Researchers and developers from around the world contributed to the project, sharing their models, insights, and codebases. This collective effort accelerated the development of AI tools designed to help healthcare professionals and policymakers make informed decisions based on up-to-date information.
Impact: The CORD-19 project led to the creation of numerous machine learning models that helped researchers quickly analyze and understand critical aspects of the pandemic. It demonstrated the power of open science and international collaboration in times of crisis, showing how the ML community can mobilize rapidly to tackle global challenges.
3. Wildbook: AI for Wildlife Conservation
Wildbook is a collaborative machine learning initiative aimed at wildlife conservation. Developed by Wild Me, an organization that combines AI and citizen science, Wildbook uses computer vision algorithms to identify and track individual animals based on their unique physical features. The project has transformed wildlife research by enabling scientists to monitor endangered species and their habitats without invasive tagging methods.
How Collaboration Fueled Success:
- Partnerships with Conservationists: Wildbook works closely with wildlife conservationists, field biologists, and environmental organizations to gather and annotate image data of animals in the wild. By partnering with experts in the field, the project ensures that the data collected is accurate and relevant to ongoing conservation efforts.
- Crowdsourced Data Collection: The platform encourages citizen scientists and wildlife enthusiasts to upload images of animals they encounter in the wild. This crowdsourcing approach has led to the creation of massive, diverse datasets, increasing the accuracy and scalability of the ML models used to identify and track animals.
- Open-Source Technology: Wildbook’s open-source nature allows developers and researchers worldwide to contribute to the project by improving algorithms, adding new features, or integrating additional species into the platform. This global collaboration has accelerated the development of cutting-edge computer vision models that are tailored to wildlife conservation.
Impact: Wildbook has been successfully used to monitor and protect endangered species such as whale sharks, giraffes, and cheetahs. By automating the identification process, the platform enables conservationists to track animal populations, study migration patterns, and detect threats, all while minimizing human intervention in natural habitats. Wildbook’s success highlights how collaborative machine learning can be harnessed for environmental and conservation purposes, helping to preserve biodiversity worldwide.
4. The Cancer Genome Atlas (TCGA)
The Cancer Genome Atlas (TCGA) is a groundbreaking collaborative effort that has transformed cancer research through the power of big data and machine learning. The TCGA project is a joint initiative by the National Cancer Institute (NCI) and the National Human Genome Research Institute (NHGRI). It has gathered molecular data on over 20,000 primary cancer and matched normal samples, encompassing more than 30 different types of cancer. Machine learning models have been applied to this data to discover patterns that could lead to new treatments and diagnostic methods.
How Collaboration Fueled Success:
- Cross-Institutional Research: TCGA involves collaboration between multiple research institutions, universities, and hospitals worldwide. By pooling resources and expertise, researchers have been able to gather and analyze a massive dataset that would have been impossible to compile individually.
- Shared Data and Open Access: The project’s data is made freely available to researchers globally, encouraging the development of new machine learning models and analytical tools that can identify genetic patterns linked to cancer. This openness has led to a diverse range of studies, each building upon the work of others.
- Interdisciplinary Approach: TCGA’s success is due to its interdisciplinary nature, combining the expertise of geneticists, oncologists, data scientists, and AI researchers. This collaborative environment fosters innovation, leading to the development of advanced ML models capable of predicting patient outcomes, personalizing treatments, and identifying new drug targets.
Impact: TCGA has led to numerous breakthroughs in understanding the genetic basis of cancer, enabling the development of personalized medicine approaches that tailor treatments to the genetic profile of each patient’s tumor. The project’s success shows how collaborative efforts that combine expertise across disciplines can revolutionize medical research and improve patient outcomes.
5. Google’s DeepMind and AlphaFold
AlphaFold, an AI system developed by Google’s DeepMind, represents a major breakthrough in protein folding—a complex problem in biology that has puzzled scientists for decades. AlphaFold’s ability to predict the 3D structure of proteins has the potential to accelerate drug discovery, improve our understanding of diseases, and create new biotechnological solutions.
How Collaboration Fueled Success:
- Partnerships with Research Institutions: DeepMind collaborated with major scientific institutions, including EMBL’s European Bioinformatics Institute (EMBL-EBI), to access the datasets and expertise necessary to train AlphaFold’s models. By working with leading researchers in structural biology, DeepMind was able to refine its algorithms to achieve unprecedented accuracy.
- Open Science and Sharing Models: In an effort to accelerate scientific progress, DeepMind made AlphaFold’s models and predictions freely available to researchers around the world. This open approach has allowed other scientists to build upon AlphaFold’s discoveries and apply its models to new areas, such as understanding the structure of viral proteins or developing new enzymes.
- Collaborative Challenges: DeepMind participated in the Critical Assessment of protein Structure Prediction (CASP) challenge, an international competition where researchers test their protein folding models against real-world data. This collaborative, competitive environment spurred innovation and helped DeepMind achieve a breakthrough with AlphaFold.
Impact: AlphaFold’s success demonstrates how collaborative efforts can solve long-standing scientific problems. By making its models and findings open-source, DeepMind has empowered the global scientific community to apply these insights to a range of challenges, from drug development to genetic engineering.
Conclusion
Collaborative machine learning projects have the power to drive innovation, solve complex problems, and create lasting impact across industries. From the groundbreaking advances of ImageNet and AlphaFold to the conservation efforts of Wildbook, these success stories highlight how collaboration can amplify the potential of machine learning. By combining expertise, sharing resources, and fostering open environments, these projects have achieved results that extend far beyond the capabilities of any single individual or organization.
Whether you are an individual data scientist, a tech company, or an academic institution, building collaborative networks and participating in open-source initiatives can lead to breakthroughs that not only advance technology but also make a positive difference in the world.
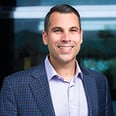
Ken Pomella
Ken Pomella is a seasoned technologist and distinguished thought leader in artificial intelligence (AI). With a rich background in software development, Ken has made significant contributions to various sectors by designing and implementing innovative solutions that address complex challenges. His journey from a hands-on developer to an entrepreneur and AI enthusiast encapsulates a deep-seated passion for technology and its potential to drive change in business.
Ready to start your data and AI mastery journey?
Visit our Teachable micro-site to explore our courses and take the first step towards becoming a data expert.