LLMs in 2024: Milestones and Future Directions
Large Language Models Dec 25, 2024 9:00:00 AM Ken Pomella 5 min read
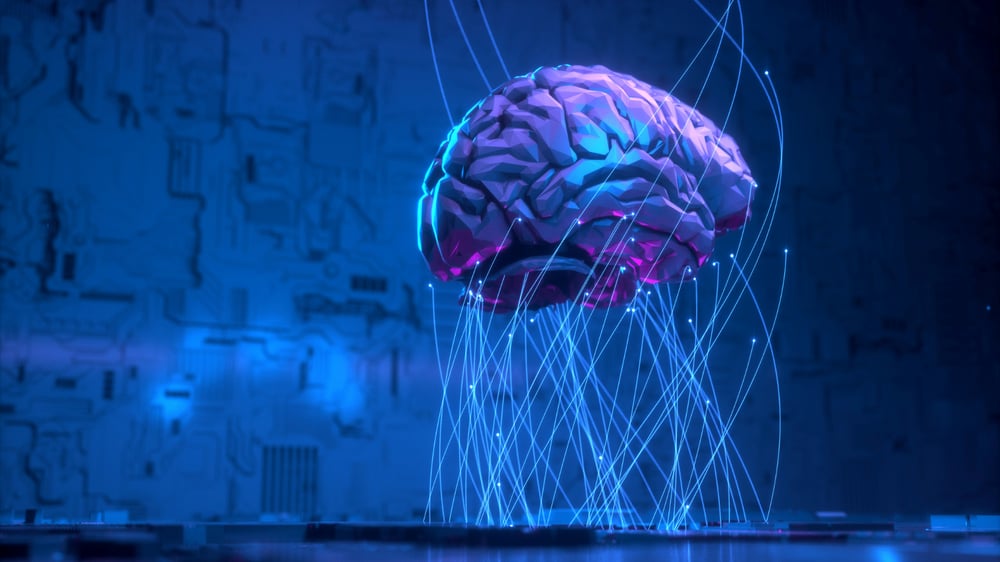
Large Language Models (LLMs) have become a cornerstone of artificial intelligence (AI), redefining how we interact with technology, process information, and solve problems. In 2024, LLMs continued to push the boundaries of natural language processing (NLP) and machine learning (ML), achieving significant milestones while charting new directions for the future.
This blog explores the key achievements of LLMs in 2024, the impact they have had across industries, and what the future holds for this transformative technology.
Milestones of LLMs in 2024
1. Multi-Modal Mastery
One of the most notable advancements in LLMs this year was their evolution into multi-modal models—AI systems capable of processing and generating content across multiple formats such as text, images, audio, and video.
Highlights:
- GPT-Vision (GPT-V): OpenAI introduced GPT-V, an extension of its GPT line that integrates visual capabilities, enabling it to analyze images, create visuals from text prompts, and even generate videos.
- DeepMind’s Gemini: Google’s DeepMind launched Gemini, a multi-modal LLM that seamlessly bridges text, images, and voice commands. Gemini powers next-generation AI assistants capable of contextual reasoning across various input types.
Impact: These advancements have opened new possibilities for applications in healthcare (diagnostic image analysis), education (interactive learning content), and content creation (AI-driven design and animation).
2. Generative AI at Scale
LLMs like ChatGPT, Claude, and others have become integral to business operations. In 2024, enterprises saw widespread adoption of customized generative AI solutions, thanks to advancements in fine-tuning and model compression.
Highlights:
- Enterprise AI on Amazon Bedrock: AWS enabled businesses to customize foundation models for specific use cases, such as customer support, supply chain optimization, and personalized marketing.
- Industry-Specific LLMs: Specialized LLMs trained for domains like law, medicine, and finance delivered more accurate and context-aware outputs than their general-purpose counterparts.
Impact: Generative AI has become more accessible and relevant for businesses, allowing them to tailor solutions to their unique challenges and workflows while reducing costs.
3. Improved Efficiency and Sustainability
The environmental impact of training and deploying large-scale models has been a growing concern. In 2024, the focus shifted to improving efficiency and sustainability in LLM development.
Highlights:
- Sparse Models: Research into sparse neural networks led to models requiring fewer computational resources without sacrificing performance. Projects like OpenAI’s SparseGPT demonstrated significant efficiency gains.
- Energy-Efficient Training: Companies like Microsoft and Google leveraged renewable energy-powered data centers and innovative training techniques to reduce carbon footprints.
Impact: By prioritizing efficiency, the AI community is addressing sustainability concerns while making LLMs more cost-effective for smaller organizations.
4. Regulation and Ethical Development
2024 witnessed significant strides in addressing the ethical and regulatory challenges posed by LLMs. Governments and organizations collaborated to establish guidelines ensuring the responsible use of AI.
Highlights:
- EU AI Act Compliance: LLM providers adopted explainability and fairness mechanisms to comply with stricter European Union regulations on AI.
- Bias Audits: Tools like Hugging Face’s FairML were used to detect and mitigate bias in language models, ensuring more equitable outcomes.
Impact: These advancements have fostered trust in LLMs, particularly in sensitive applications like hiring, lending, and law enforcement, where ethical concerns are paramount.
5. Real-Time Learning and Adaptability
2024 marked significant progress in real-time learning, enabling LLMs to stay updated with the latest information and adapt to changing contexts dynamically.
Highlights:
- Continuous Learning Pipelines: LLMs now incorporate mechanisms for incremental updates, reducing the need for complete retraining when new data becomes available.
- Real-Time Fact-Checking: Integrated fact-checking systems ensure that LLM outputs remain accurate and reliable, addressing concerns around misinformation.
Impact: Real-time adaptability has enhanced the reliability of LLMs in areas such as journalism, legal research, and dynamic customer support.
Future Directions for LLMs
1. Deeper Personalization
The next generation of LLMs is expected to deliver highly personalized interactions, tailoring responses based on individual preferences, behavior, and context.
What to Expect:
- Personal AI assistants that adapt to users’ evolving needs, managing tasks, scheduling, and even maintaining long-term context for better recommendations.
- Enhanced user control over data and customization, ensuring privacy and transparency in how personal information is used.
2. Integration with Emerging Technologies
LLMs are set to play a pivotal role in advancing other cutting-edge technologies, from augmented reality (AR) to quantum computing.
Potential Applications:
- AR/VR Experiences: LLMs could enable conversational interfaces within immersive environments, revolutionizing gaming, virtual shopping, and training simulations.
- Quantum ML: As quantum computing matures, LLMs trained on quantum-accelerated architectures could achieve unprecedented levels of efficiency and capability.
3. Interoperability and Collaboration
Future LLMs will focus on collaboration across systems, enabling seamless integration with other AI models, platforms, and enterprise tools.
What to Expect:
- Open standards for LLM interoperability, allowing businesses to use multiple models within a single ecosystem.
- Collaboration between LLMs and specialized models for tasks like image recognition, real-time translation, and predictive analytics.
4. Edge Deployment and Offline Functionality
As demand for on-device AI grows, LLMs are likely to become more compact and efficient, enabling deployment on edge devices without relying on cloud infrastructure.
Potential Applications:
- AI-powered personal devices like smart assistants, AR glasses, and autonomous vehicles with low-latency, offline capabilities.
- Enhanced security and privacy for sensitive data, processed locally instead of being transmitted to the cloud.
5. Expanding Accessibility and Inclusion
The future of LLMs will focus on breaking down barriers to ensure global accessibility and inclusivity.
What to Expect:
- Multilingual Models: LLMs supporting underrepresented languages and dialects will enable broader access to AI-driven tools worldwide.
- Simplified Interfaces: Continued improvements in natural language interfaces will make LLMs more accessible to non-technical users.
Conclusion: The Evolving Role of LLMs
In 2024, LLMs reached new heights of innovation, driving change across industries and setting the stage for even greater advancements. From multi-modal capabilities to real-time adaptability and ethical governance, LLMs are becoming indispensable tools for businesses, researchers, and consumers alike.
As we look ahead, the potential for LLMs to shape the future is limitless. By focusing on personalization, interoperability, and inclusion, the next wave of LLMs promises to make AI not just smarter, but more human-centric and accessible for everyone.
The journey of LLMs is far from over. With each breakthrough, they are bringing us closer to a future where technology seamlessly augments every aspect of our lives—responsibly, creatively, and inclusively.
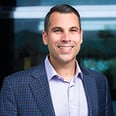
Ken Pomella
Ken Pomella is a seasoned technologist and distinguished thought leader in artificial intelligence (AI). With a rich background in software development, Ken has made significant contributions to various sectors by designing and implementing innovative solutions that address complex challenges. His journey from a hands-on developer to an entrepreneur and AI enthusiast encapsulates a deep-seated passion for technology and its potential to drive change in business.
Ready to start your data and AI mastery journey?
Visit our Teachable micro-site to explore our courses and take the first step towards becoming a data expert.