Ethical AI: Machine Learning with Responsibility
Machine Learning AI Ethics Oct 16, 2024 9:00:00 AM Ken Pomella 6 min read
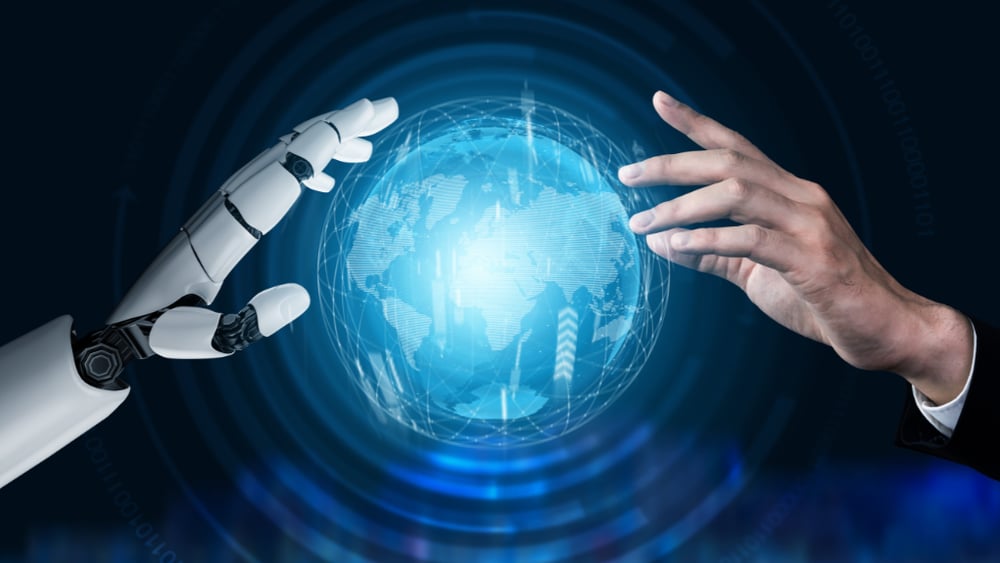
As artificial intelligence (AI) and machine learning (ML) continue to advance, they are increasingly woven into the fabric of our everyday lives—from personalized recommendations on streaming platforms to predictive models in healthcare. While AI holds enormous potential for innovation and positive impact, its widespread use also raises critical ethical questions. Ensuring that AI is developed and deployed responsibly is no longer optional; it’s essential for building trust, avoiding harm, and promoting fairness.
In this blog, we explore the key principles of Ethical AI, the challenges associated with responsible machine learning, and the steps organizations can take to ensure that AI is used ethically.
What is Ethical AI?
Ethical AI refers to the development and use of artificial intelligence systems in ways that align with widely accepted ethical principles, including fairness, accountability, transparency, and the prevention of harm. The goal of ethical AI is to ensure that AI technologies are designed and implemented in ways that are safe, equitable, and respectful of human rights.
Why Ethical AI Matters
As machine learning models become more powerful and complex, they increasingly affect decision-making in critical areas such as hiring, healthcare, law enforcement, and financial services. While AI can provide efficiencies and insights, it can also introduce risks such as:
- Bias and Discrimination: AI systems can perpetuate or amplify biases present in their training data, leading to unfair outcomes for certain groups.
- Lack of Accountability: Automated decisions made by opaque AI models, or "black-box" algorithms, can be difficult to explain or challenge.
- Privacy Concerns: The large datasets used to train AI models often contain sensitive personal information, raising concerns about how that data is collected, stored, and used.
Ensuring that AI systems adhere to ethical guidelines is critical for minimizing these risks and fostering a more responsible use of technology.
Key Ethical Principles for AI and Machine Learning
To build AI systems that are ethical and responsible, organizations should adopt the following core principles:
1. Fairness and Inclusivity
AI models should be designed to treat all individuals and groups fairly, avoiding discrimination or bias based on race, gender, age, socioeconomic status, or other characteristics. This principle is particularly important in areas where AI decisions can significantly impact people's lives, such as job hiring, lending, or criminal justice.
Challenges:
- Bias in Data: Machine learning models are only as unbiased as the data they are trained on. If the training data reflects historical inequalities, the AI model may reinforce or amplify these biases.
- Algorithmic Bias: Even without biased data, certain algorithms may disproportionately affect some groups over others.
Best Practices:
- Diverse Data Sources: Use diverse and representative datasets during model training to reduce the likelihood of introducing bias.
- Bias Audits: Conduct regular bias audits and fairness assessments to identify and mitigate potential biases in AI models.
- Algorithmic Fairness: Incorporate fairness constraints in the algorithmic design to ensure equitable outcomes across all demographic groups.
2. Transparency and Explainability
AI models should be transparent, meaning that stakeholders can understand how the AI system works, what data it uses, and why it makes specific decisions. Explainability allows users and affected individuals to trust the model and ensures accountability.
Challenges:
- Black-Box Models: Many machine learning models, especially deep learning models, are considered “black boxes” because they make predictions or decisions without easily understandable reasoning.
- Complexity vs. Explainability: There is often a trade-off between model complexity and explainability. Complex models may perform better but be harder to interpret.
Best Practices:
- Interpretable Models: Use interpretable models (e.g., decision trees, linear regression) where possible, especially in high-stakes decision-making contexts.
- Explainability Tools: For more complex models, employ post-hoc explainability tools like LIME (Local Interpretable Model-Agnostic Explanations) or SHAP (Shapley Additive Explanations) to provide insight into how the model makes decisions.
- Transparent Reporting: Develop clear documentation that outlines how models were trained, what data was used, and how decisions are made.
3. Accountability
It is crucial to assign responsibility for the outcomes generated by AI systems. AI systems should not be autonomous in ways that absolve humans of responsibility, especially when these systems are making decisions that affect people's lives.
Challenges:
- Diffuse Accountability: In complex AI ecosystems, it can be difficult to assign responsibility for an algorithm’s actions, particularly in large organizations where multiple teams may be involved in its development and deployment.
- Legal and Regulatory Gaps: Current legal frameworks may not fully address the accountability challenges posed by AI systems.
Best Practices:
- Clear Ownership: Assign clear ownership and accountability for the development, deployment, and monitoring of AI systems within the organization.
- Human-in-the-Loop: For high-impact decisions, ensure that humans remain involved in the decision-making process, particularly to validate or override AI-driven recommendations.
- Audits and Reviews: Conduct regular internal and external audits of AI systems to ensure they comply with ethical guidelines and industry regulations.
4. Privacy and Data Protection
AI systems often rely on large volumes of data, which may include sensitive personal information. It is essential to protect individuals' privacy by ensuring that data is collected, stored, and used responsibly.
Challenges:
- Data Collection: The data required to train AI models can include personal or sensitive information, raising concerns about how this data is obtained and whether individuals have consented to its use.
- Data Breaches: AI systems are not immune to cyberattacks, and the large datasets they use can be attractive targets for hackers.
Best Practices:
- Data Minimization: Collect only the data necessary for the specific AI application. Avoid using or storing personal data unless it is essential.
- Data Anonymization: Apply data anonymization techniques to protect individuals’ identities in the dataset.
- Comply with Regulations: Ensure that your AI systems comply with data protection laws such as GDPR (General Data Protection Regulation) or CCPA (California Consumer Privacy Act).
5. Avoiding Harm
AI systems should be designed to prevent harm, both directly and indirectly, to individuals or groups. This principle is particularly relevant in fields like healthcare, law enforcement, and finance, where AI decisions can have significant consequences.
Challenges:
- Unintended Consequences: AI models can sometimes produce unexpected outcomes that may inadvertently harm certain individuals or groups.
- Automation Risks: Automated systems that make critical decisions—such as predicting medical outcomes or approving loans—can lead to serious consequences if errors go unnoticed.
Best Practices:
- Rigorous Testing: Before deploying AI systems, conduct extensive testing to ensure the model’s decisions do not lead to harmful outcomes. Simulate different scenarios to evaluate the robustness of the model.
- Continuous Monitoring: Even after deployment, AI systems should be monitored continuously to detect and address any harmful behavior.
- Ethical Impact Assessments: Regularly assess the potential societal and individual impacts of your AI systems to ensure they do not contribute to harmful outcomes.
Building a Framework for Ethical AI
To implement ethical AI practices effectively, organizations need to build a strong ethical framework that guides the development and deployment of AI systems. Here are some steps to create such a framework:
- Establish Ethical AI Guidelines: Develop a set of ethical AI principles that align with your organization’s values and societal responsibilities. These principles should cover fairness, transparency, accountability, and privacy.
- Cross-Functional Collaboration: Form cross-functional teams that include not only AI engineers but also legal, compliance, and ethics experts. This ensures that ethical considerations are built into the design and implementation of AI systems.
- Train Employees on AI Ethics: Offer regular training on AI ethics to ensure that all employees, especially those involved in AI development and deployment, are aware of ethical risks and best practices.
- Create an Ethics Review Board: Establish an internal ethics review board to oversee AI projects, ensuring that they adhere to ethical guidelines and assessing potential risks before deployment.
- Engage with the AI Ethics Community: Participate in the broader AI ethics community to stay updated on emerging ethical challenges and best practices. Collaborate with other organizations, academic institutions, and policy makers to contribute to the development of responsible AI.
Conclusion
As AI and machine learning continue to transform industries, the importance of ethical AI cannot be overstated. Building machine learning systems with responsibility requires a deep commitment to fairness, transparency, accountability, and privacy. Organizations that prioritize these ethical principles will not only avoid the pitfalls of biased or harmful AI but also foster trust with users, customers, and the public.
By incorporating best practices for ethical AI into your organization’s strategy, you can ensure that your machine learning systems are designed and deployed in ways that benefit society while minimizing risks. The future of AI is bright, but it must be guided by responsibility and ethical considerations to truly realize its potential for good.
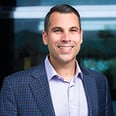
Ken Pomella
Ken Pomella is a seasoned technologist and distinguished thought leader in artificial intelligence (AI). With a rich background in software development, Ken has made significant contributions to various sectors by designing and implementing innovative solutions that address complex challenges. His journey from a hands-on developer to an entrepreneur and AI enthusiast encapsulates a deep-seated passion for technology and its potential to drive change in business.
Ready to start your data and AI mastery journey?
Visit our Teachable micro-site to explore our courses and take the first step towards becoming a data expert.