Data Engineering Trends for 2025: What You Need to Know
Tech Industry Trends Real-Time Analytics Data Jan 15, 2025 9:00:00 AM Ken Pomella 4 min read
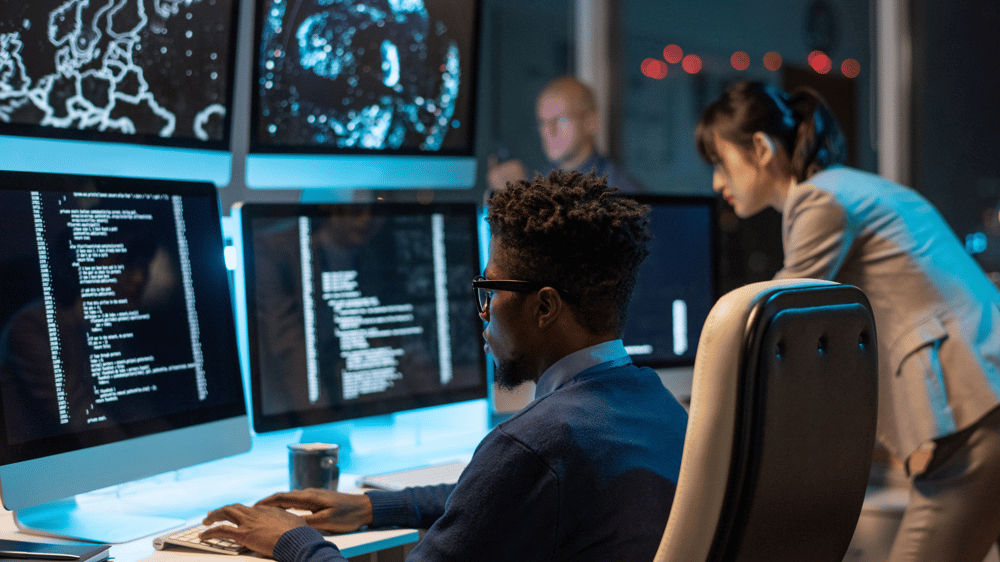
Data engineering is the backbone of the modern data ecosystem, empowering organizations to store, process, and analyze massive amounts of information efficiently. In 2025, this critical field is evolving rapidly, driven by emerging technologies, increased demand for real-time insights, and the growing adoption of AI and machine learning.
For data professionals, keeping up with these trends is essential for staying competitive and delivering value in a data-driven world. This blog explores the key data engineering trends shaping 2025 and what you need to know to stay ahead.
Real-Time Data Processing Takes Center Stage
In 2025, the ability to process data in real time is no longer a luxury—it’s a necessity. Businesses across industries are demanding faster insights to power decision-making, enhance customer experiences, and optimize operations.
Why It Matters:
Real-time data processing enables companies to respond to events as they happen, such as detecting fraudulent transactions, personalizing customer interactions, or optimizing supply chains.
How to Prepare:
- Learn tools like Apache Kafka, Apache Flink, and AWS Kinesis, which are leading solutions for real-time data streaming.
- Develop expertise in event-driven architectures, which are critical for designing systems that handle real-time data efficiently.
The Rise of Data Mesh
Traditional centralized data architectures struggle to scale with the increasing complexity of modern organizations. In 2025, data mesh is emerging as a game-changing approach, decentralizing data ownership and enabling teams to manage their own data domains.
Why It Matters:
Data mesh improves scalability and agility by allowing teams to access and analyze data independently while maintaining governance and quality across the organization.
How to Prepare:
- Understand the principles of data mesh, such as domain-oriented ownership, self-serve data infrastructure, and federated governance.
- Explore tools that support data mesh implementations, such as Snowflake, Databricks, and dbt.
Cloud-Native Data Engineering Dominates
The shift to the cloud continues to accelerate, with cloud-native data engineering tools becoming the default choice for organizations. In 2025, businesses are fully embracing cloud platforms for their scalability, flexibility, and cost-effectiveness.
Why It Matters:
Cloud-native solutions reduce the complexity of managing infrastructure while enabling organizations to scale resources dynamically based on demand.
How to Prepare:
- Gain expertise in cloud platforms such as AWS, Google Cloud, and Microsoft Azure.
Master cloud-based tools like BigQuery, Redshift, and Azure Synapse for data warehousing and analytics. - Familiarize yourself with containerization technologies like Docker and orchestration platforms like Kubernetes for cloud-native deployments.
Automation and DataOps
Automation is transforming data engineering workflows in 2025, with DataOps emerging as a key trend. By applying DevOps principles to data engineering, DataOps focuses on improving collaboration, automating repetitive tasks, and delivering high-quality data pipelines faster.
Why It Matters:
DataOps enhances productivity, reduces errors, and accelerates the delivery of data insights to business stakeholders.
How to Prepare:
- Learn CI/CD (Continuous Integration and Continuous Deployment) practices for data engineering workflows.
- Explore tools like Apache Airflow, Prefect, and Dagster for pipeline orchestration and monitoring.
- Understand the importance of data observability and adopt tools like Monte Carlo and Great Expectations.
AI-Powered Data Engineering
AI and machine learning are reshaping how data engineering is performed, automating complex tasks such as schema mapping, anomaly detection, and data quality monitoring.
Why It Matters:
AI-driven tools reduce manual effort and improve the accuracy and efficiency of data engineering processes, freeing up engineers to focus on higher-value tasks.
How to Prepare:
- Explore AI-powered tools like DataRobot, AWS Glue, and Databricks AutoML.
- Learn how to integrate AI capabilities into your workflows, such as using machine learning for predictive maintenance of data pipelines.
Emphasis on Data Security and Governance
With growing concerns about data privacy and stricter regulations, organizations in 2025 are prioritizing data security and governance more than ever.
Why It Matters:
Compliance with regulations like GDPR, CCPA, and the upcoming AI governance frameworks is critical for avoiding legal and reputational risks.
How to Prepare:
- Master data governance frameworks and tools like Collibra, Alation, and AWS Lake Formation.
- Stay updated on emerging regulations and best practices for data privacy and security.
- Implement robust access controls and encryption mechanisms to protect sensitive data.
Sustainability in Data Engineering
As organizations increasingly commit to sustainability goals, data engineering in 2025 is focusing on reducing the environmental impact of data processing and storage.
Why It Matters:
Efficient data engineering practices contribute to lower energy consumption, aligning with corporate sustainability initiatives and reducing operational costs.
How to Prepare:
- Optimize data storage and processing by using scalable solutions like Snowflake and BigQuery, which offer efficient resource usage.
- Learn techniques for reducing data redundancy and improving energy efficiency in data workflows.
- Explore cloud providers’ sustainability efforts, such as AWS’s Sustainability Pillar in the Well-Architected Framework.
Edge Computing and IoT
Edge computing is gaining traction in 2025 as the Internet of Things (IoT) expands, enabling data to be processed closer to its source.
Why It Matters:
Edge computing reduces latency, enhances real-time decision-making, and minimizes the bandwidth required to transmit data to central servers.
How to Prepare:
- Familiarize yourself with edge computing frameworks like AWS IoT Greengrass, Google Edge TPU, and Azure IoT Edge.
- Develop skills in managing distributed data systems and integrating IoT devices into data pipelines.
Conclusion
Data engineering in 2025 is marked by exciting advancements and new challenges. From real-time data processing and AI-driven automation to data mesh and cloud-native architectures, the field is evolving rapidly. By staying informed about these trends and upskilling in critical areas, you can position yourself as a leader in the data engineering space.
Prepare for the future by focusing on the skills, tools, and best practices shaping the industry, and take advantage of the opportunities presented by these transformative trends.
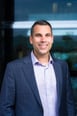
Ken Pomella
Ken Pomella is a seasoned software engineer and a distinguished thought leader in the realm of artificial intelligence (AI). With a rich background in software development, Ken has made significant contributions to various sectors by designing and implementing innovative solutions that address complex challenges. His journey from a hands-on developer to an AI enthusiast encapsulates a deep-seated passion for technology and its potential to drive change.
Ready to start your data and AI mastery journey?
Visit our Teachable micro-site to explore our courses and take the first step towards becoming a data expert.